History of AI
1950s-1960s:This era is marked by the development of symbolic
AI, where researchers focused on creating systems capable of reasoning
and problem-solving using symbolic manipulation. Early projects during
this time include the Logic Theorist, General Problem Solver (GPS),
and the first AI program to play chess.
1970s-1980s: Symbolic AI faced challenges in dealing with
uncertainty and complexity, leading to the emergence of new approaches
such as expert systems and knowledge-based systems. Expert systems
attempted to encode human knowledge and expertise into rule-based
systems.
Late 20th Century: The field of AI experienced periods of both
optimism and skepticism, known as AI summers and winters,
respectively. AI winters were characterized by reduced funding and
interest due to unmet expectations and limited progress.
1990s-Present: Advances in computing power, algorithms, and
data availability have led to significant progress in AI. Machine
learning, particularly deep learning, has revolutionized the field by
enabling systems to learn from data rather than being explicitly
programmed.
21st Century: AI applications have become pervasive across
various domains, including healthcare, finance, transportation, and
entertainment. Breakthroughs in natural language processing (NLP),
computer vision, and reinforcement learning have led to the
development of intelligent systems capable of human-level performance
in certain tasks.
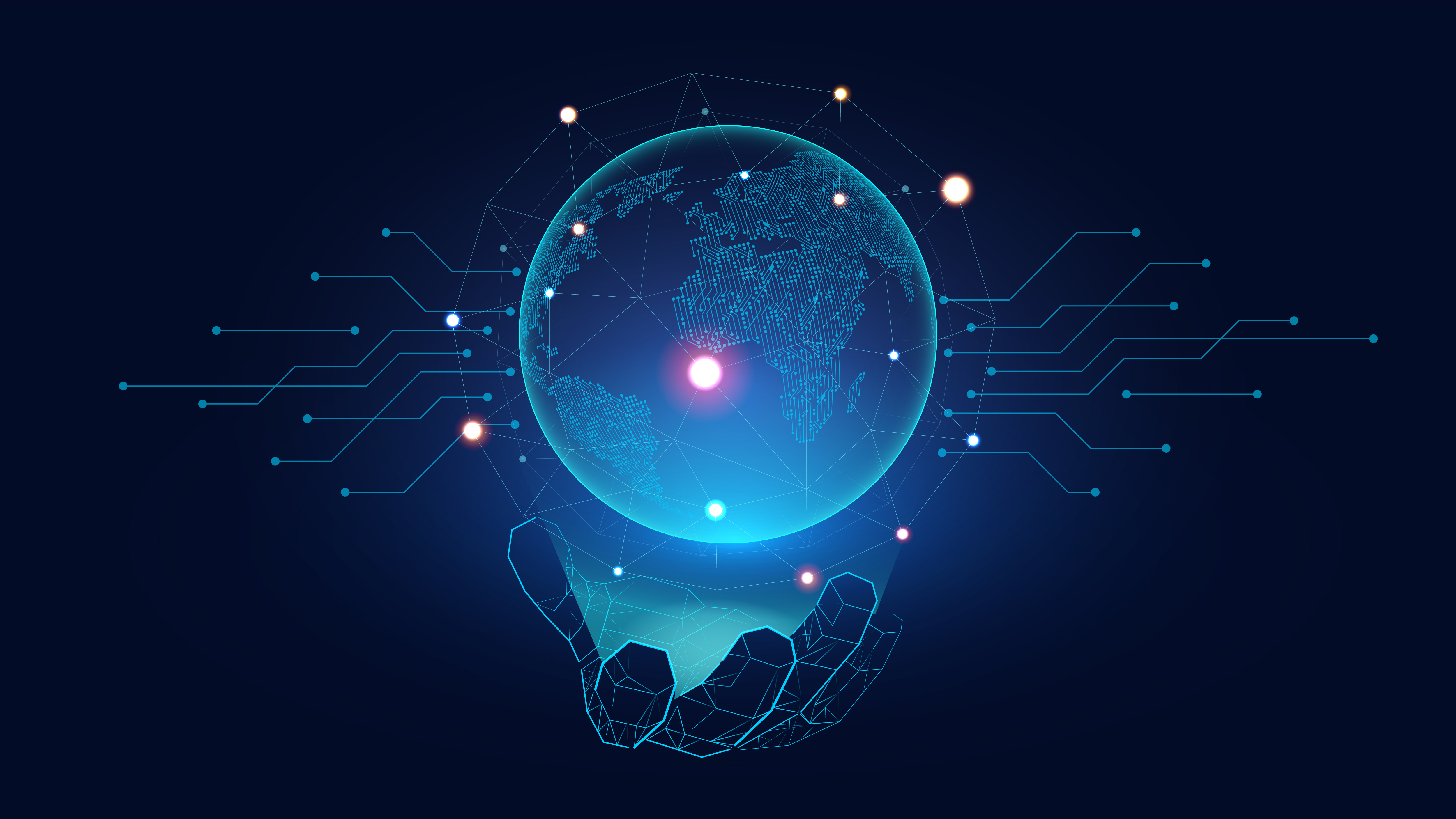
The three kinds of AI based on capabilities
1. Artificial Narrow AI Artificial Narrow Intelligence, also
known as Weak AI, what we refer to as Narrow AI is the only type of AI
that exists today. Any other form of AI is theoretical. It can be
trained to perform a single or narrow task, often far faster and
better than a human mind can. However, it can’t perform outside of its
defined task.
2. General AI Artificial General Intelligence (AGI), also known
as Strong AI, is today nothing more than a theoretical concept. AGI
can use previous learnings and skills to accomplish new tasks in a
different context without the need for human beings to train the
underlying models.
3. Super AI Super AI is commonly referred to as artificial
superintelligence and, like AGI, is strictly theoretical. If ever
realized, Super AI would think, reason, learn, make judgements and
possess cognitive abilities that surpass those of human beings.
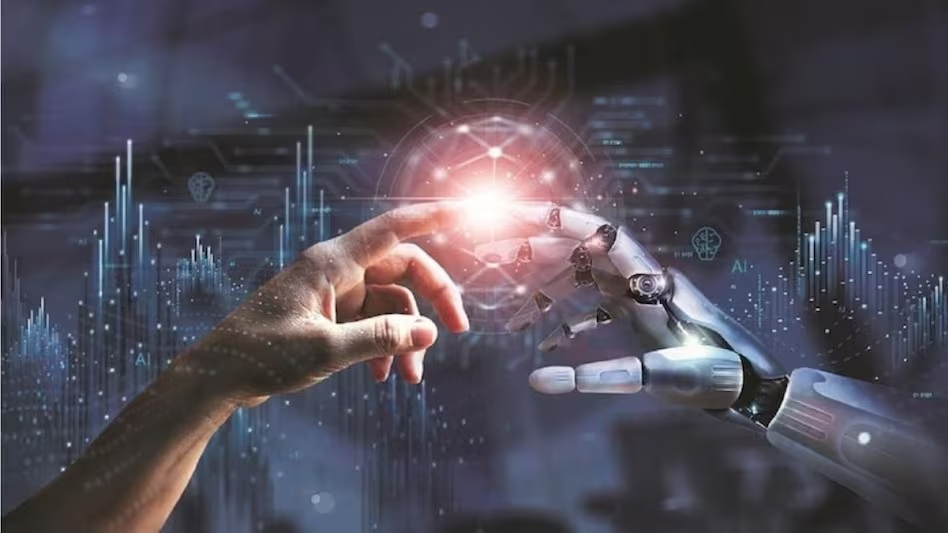
The four types of AI based on functionalities
1. Reactive Machine AI:-
Reactive machines are AI systems with no memory and are designed to
perform a very specific task. Since they can’t recollect previous
outcomes or decisions, they only work with presently available data.
Reactive AI stems from statistical math and can analyze vast amounts
of data to produce a seemingly intelligence output.
2. Limited Memory AI:-
Unlike Reactive Machine AI, this form of AI can recall past events and
outcomes and monitor specific objects or situations over time. Limited
Memory AI can use past- and present-moment data to decide on a course
of action most likely to help achieve a desired outcome. However,
while Limited Memory AI can use past data for a specific amount of
time, it can’t retain that data in a library of past experiences to
use over a long-term period.
3. Theory of Mind AI:-
Theory of Mind AI is a functional class of AI that falls underneath
the General AI. Though an unrealized form of AI today, AI with Theory
of Mind functionality would understand the thoughts and emotions of
other entities. This understanding can affect how the AI interacts
with those around them. In theory, this would allow the AI to simulate
human-like relationships.
4. Self-Aware AI:-
Self-Aware AI is a kind of functional AI class for applications that
would possess super AI capabilities. Like theory of mind AI,
Self-Aware AI is strictly theoretical. If ever achieved, it would have
the ability to understand its own internal conditions and traits along
with human emotions and thoughts. It would also have its own set of
emotions, needs and beliefs.
Additional capabilities and practical applications of AI technologies.....
Computer vision:-
Narrow AI applications with computer vision can be trained to interpret and analyze the visual world. This allows intelligent machines to identify and classify objects within images and video footage.
Applications of computer vision include:
Image recognition and classification
Object detection
Object tracking
Facial recognition
Content-based image retrieval
Computer vision is critical for use cases that involve AI machines interacting and traversing the physical world around them. Examples include self-driving cars and machines navigating warehouses and other environments.
Robotics:-
Robots in industrial settings can use Narrow AI to perform routine, repetitive tasks that involve materials handling, assembly and quality inspections. In healthcare, robots equipped with Narrow AI can assist surgeons in monitoring vitals and detecting potential issues during procedures. Agricultural machines can engage in autonomous pruning, moving, thinning, seeding and spraying. And smart home devices such as the iRobot Roomba can navigate a home’s interior using computer vision and use data stored in memory to understand its progress.
Expert systems:-
Expert systems equipped with Narrow AI capabilities can be trained on a corpus to emulate the human decision-making process and apply expertise to solve complex problems. These systems can evaluate vast amounts of data to uncover trends and patterns to make decisions. They can also help businesses predict future events and understand why past events occurred.